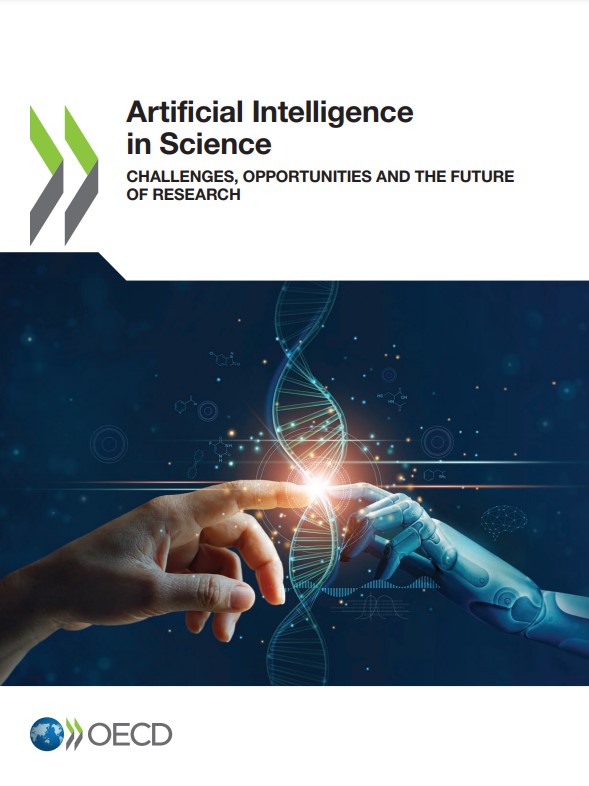
□ OECD는 진보가 필요한 과학에 대한 ‘인공지능과 과학 : 도전과제, 기회, 그리고 미래 연구’ 보고서를 발간함
ㅇ 인공지능(AI)을 과학 분야에 활용하고 연구에 대한 긍정적인 기여를 강화하기 위해서는 정책 결정자 및 연구시스템 차원에서의 노력이 필요
- AI의 과학 분야 활용은 혁신을 통한 경제적 영향이 크며 생산성 향상 등을 통해 성장 효과가 장기간 지속될 수 있음
- 기존 경제 성장률 유지를 위해 필요한 노력이 증가하는 상황에서 과학이 점차 어려워진다는 견해가 있으며, AI는 이러한 어려움을 다방면으로 보조 가능
※ 기후 모델링, 세포 연구, 알츠하이머 등 여전히 과학적 지식이 부족한 분야들이 존재하며, AI는 연구와 해결책의 탐색 모두에 도움이 될 수 있음
ㅇ 과학 분야 AI는 현재 실험 설계, 가설 설정, 시뮬레이션 등 연구 진행 과정에 폭넓게 기여하고 있으나, 다학제 간 협력을 통한 AI 모델 개발 노력이 부족
- 머신러닝에 기반한 AI 뿐만 아니라 새로운 생성형 AI의 도입은 데이터의 품질을 개선하고 저비용으로 수집된 데이터의 정밀도를 높일 수 있음
※ 생성형 AI 모델은 전통적인 알고리즘과 다르게 오작동 될 수 있으므로, 편견/편향을 어떻게 조절하는지가 중요한 문제임
- 좋은 AI 모델 개발을 위해서는 다학제 연구가 필요하지만 지나친 경쟁으로 협력이 어려워지고, 민간영역의 AI 연구 주제가 더 협소하거나 전문화됨
□ 보고서는 AI의 발전을 위한 다양한 주제를 다루고 있으며 본 동향에서는 연구 거버넌스, 다학제 프로그램, 공공 R&D의 역할을 소개
(출처: 한국과학기술기획평가원)
[관련 자료] 「OECD Artificial intelligence in science: challenges, opportunities and the future of research」의 주요 내용 및 시사점 (2023.09.20.)
/ 한국과학기술기획평가원
목차
Preface 3
Foreword 4
Acknowledgements 5
Executive summary 10
Artificial intelligence in science: Overview and policy proposals
by Alistair Nolan 13
Part I Is science getting harder? 49
Are ideas getting harder to find? A short review of the evidence
by Matt Clancy 51
The end of Moore’s Law? Innovation in computer systems continues at a high pace
by Henry Kressel 58
Is technological progress in US agriculture slowing?
by Matt Clancy 62
Eroom’s Law and the decline in the productivity of biopharmaceutical R&D
by Jack W. Scannell 70
Is there a slowdown in research productivity? Evidence from China and Germany
by Philipp Boeing and Paul Hünermund 79
Declining R&D efficiency: Evidence from Japan
by Tsutomu Miyagawa 85
Quantifying the “cognitive extent” of science and how it has changed over time and
across countries
by Staša Milojević 89
What can bibliometrics contribute to understanding research productivity?
by Giovanni Abramo and Ciriaco A. D’Angelo 95
Part II Artificial intelligence in science today 101
How can artificial intelligence help scientists? A (non-exhaustive) overview
by Aishik Ghosh 103
A framework for evaluating the AI-driven automation of science
by Ross King and Hector Zenil 113
Using machine learning to verify scientific claims
by Lucy Lu Wang 121
Robot scientists: From Adam to Eve to Genesis
by Patrick Courtney, Ross King and Oliver Peter 129
From knowledge discovery to knowledge creation: How can literature-based
discovery accelerate progress in science?
by Gus Hahn-Powell, Dimitar Hristovski, Yakub Sebastian and Neil R. Smalheiser 140
Advancing the productivity of science with citizen science and artificial intelligence
by James Bibby, Luigi Ceccaroni, Paul Flemons, Alexis Joly, Katina Michael, Jessica L. Oliver and
Erin Roger 148
What can artificial intelligence do for physics?
by Sabine Hossenfelder 155
AI in drug discovery
by Kristof Z.Szalay 158
Data-driven innovation in clinical pharmaceutical research
by Joshua New 167
Applying AI to real-world health-care settings and the life sciences: Tackling data
privacy, security and policy challenges with federated learning
by Mathieu Galtier and Darius Meadon 171
Part III The near future: challenges and ways forward 179
Artificial intelligence in scientific discovery: Challenges and opportunities
by Ross King and Hector Zenil 181
Machine reading: Successes, challenges and implications for science
by Jesse Dunietz 188
Interpretability: Should – and can – we understand the reasoning of machinelearning systems?
by Hugh M. Cartwright 200
Combining collective and machine intelligence at the knowledge frontier
by Aleks Berditchevskaia and Eirini Malliaraki 206
Elicit: Language models as research tools
by Jungwon Byun and Andreas Stuhlmüller 214
Democratising artificial intelligence to accelerate scientific discovery
by Joaquin Vanschoren 224
Is there a narrowing of AI research?
by Joel Klinger and Juan Mateos-Garcia 230
Lessons from shortcomings in machine learning for medical imaging
by Veronika Cheplygina and Gaël Varoquaux 238
Part IV Artificial intelligence in science: Implications for public policy 243
Artificial intelligence for science and engineering: A priority for public investment in
research and development
by Tony Hey 245
The importance of knowledge bases for artificial intelligence in science
by Ken Forbus 251
High-performance computing leadership to enable advances in artificial intelligence
and a thriving compute ecosystem
by Mallikarjun Shankar, Georgia Tourassi and Feiyi Wang 257
Improving reproducibility of artificial intelligence research to increase trust and
productivity
by Odd Erik Gundersen 262
AI and scientific productivity: Considering policy and governance challenges
by Kieron Flanagan, Priscila F.De Oliveira and Barbara Ribeiro 271
Part V Artificial intelligence, science and developing countries 279
Artificial intelligence and development projects: A case study in funding mechanisms
to optimise research excellence in sub-Saharan Africa
by Davor Orlić and John Shawe-Taylor 281
Artificial intelligence for science in Africa
by Gregg Barrett 287
Artificial intelligence, developing-country science and bilateral co-operation
by Peter M.Addo 294
해시태그
관련자료
AI 100자 요약·번역서비스
인공지능이 자동으로 요약·번역한 내용입니다.
Artificial Intelligence in Science : challenges, opportunities and the future of research
(과학에서의 인공지능 : 도전, 기회 및 연구의 미래)